Meta's pioneering AI researcher is experimenting with the fundamental concept of artificial intelligence.
Transforming the Tone:
Hey there! Let's dive into the thoughts of Yann LeCun, the AI whiz at Meta, who's shaking things up in the AI world. He's throwing a wrench in the "Scaling Rules" that have been driving AI development for ages. These rules propose that bigger AI models equate to more power and intelligence. However, Yann doesn't quite agree.
Enrichment Data Integration:
Taking a close look at Yann LeCun's arguments, he fundamentally disagrees with the widely accepted "Scaling Laws." These laws have been shaping AI for years, as outlined in the 2020 work "Scaling Laws for Neural Language Models." Essentially, the performance of AI is believed to be highly dependent on scale—composed of three factors: the number of model parameters, the size of the dataset, and computational resources used for training.
Restructuring for Clarity:
However, Yann LeCun isn't convinced that it's always about scaling up. According to him, focusing only on scaling misses the mark in many cases.
Revise and Vary Sentences:
In essence, the AI industry has been chasing the dream that bigger models equal more intelligence, fueling massive investments in data centers. But as Yann points out during a keynote at the National University of Singapore, that's not the whole story.
Flow and Coherence:
He argues that simple AI systems might excel in basic tasks, but this success doesn't guarantee equivalent performance on complex problems. The notion that scaling these systems would lead to smarter AI is misguided. Moreover, Yann contends that expanding AI models by adding data and computational power won't necessarily lead to superintelligence.
Paragraph Adjustments:
Recently, AI progress has been sluggish, partly due to a shrinking pool of usable public data. And Yann LeCun isn't the only one questioning the power of scaling. Other influential researchers in Silicon Valley share similar concerns.
Output:
Embracing a world-based training approach, LeCun believes we need AI systems that can learn new tasks rapidly and have a better grasp of the real world: text, language, and the physical world. Furthermore, they should possess common sense, logical reasoning, and persistent memory—the hallmarks of intelligent entities.
Last year, on Lex Fridman's podcast, LeCun mentioned world models as a potential solution. Unlike traditional large language models, these world models exhibit a higher level of cognition, able to predict the consequences of actions. It's all about stepping away from mere pattern prediction and moving towards a more sophisticated understanding of the world. Don't you think?
- What's LeCun's alternative to the traditional scaling approach in AI development? He suggests the implementation of world-based training, enabling AI systems to learn new tasks swiftly, understand the real world (text, language, and the physical world), and demonstrate elements of intelligence like common sense, logical reasoning, and persistent memory.
- Recently, OpenAI has been working on a technology called 'world models' that could potentially solve the limitations of traditional large language models. World models show a higher level of cognition, as they can predict the consequences of actions, thereby stepping beyond mere pattern prediction and enhancing our understanding of the world.
- In addition, LeCun's work emphasizes the importance of intelligent embeddings in AI, which would enable AI systems to have a more sophisticated understanding of the world, surpassing the limitations of simple AI that excels only in basic tasks.
- Despite the significant investments in data centers to create larger AI models, the traditional scaling approach could be misguided when applied to complex problems. The notion that scaling AI would necessarily lead to superintelligence is a misunderstanding, as highlighted by Yann LeCun in his discussions at the National University of Singapore.
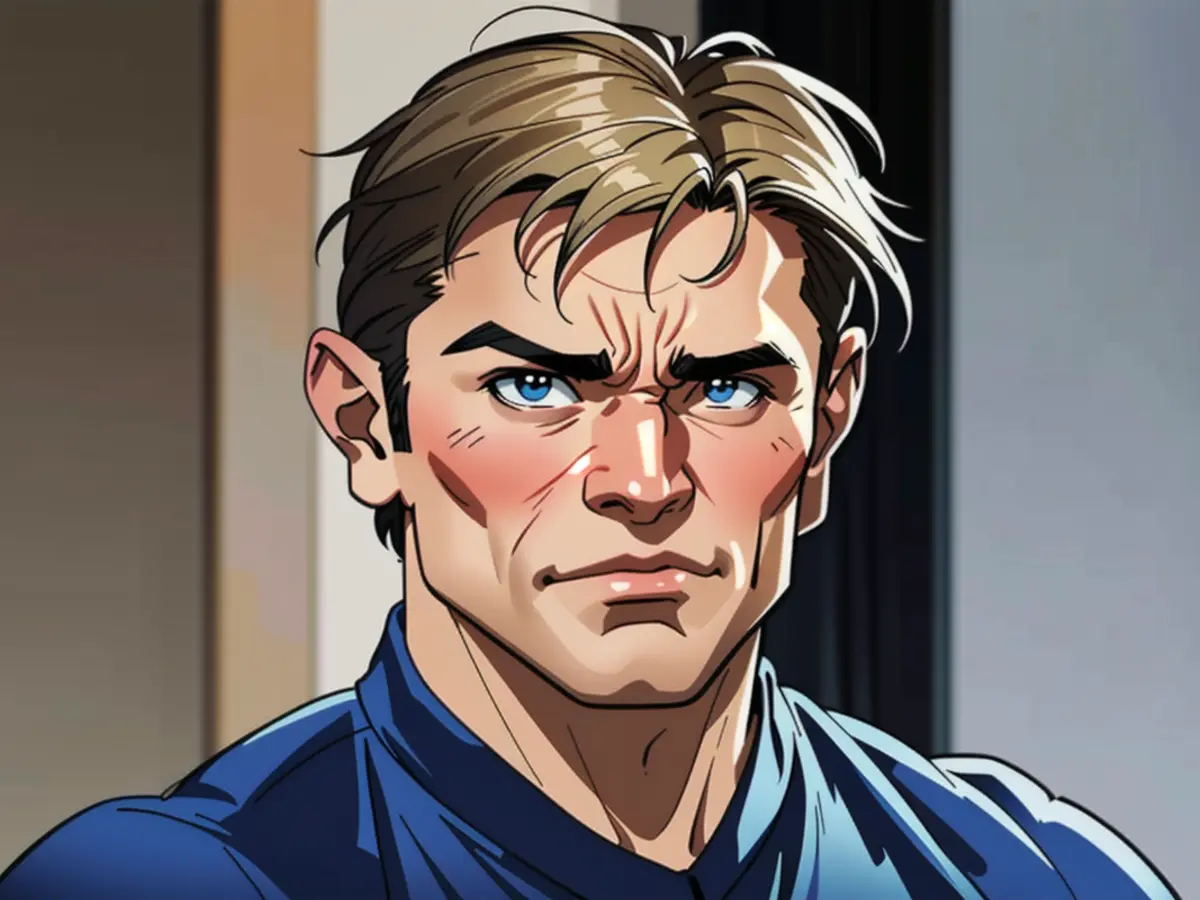